Artificial Intelligence & OSINT : Part 1
In this article from Nidal Morrison we take a closer look at how AI can be leveraged in OSINT as a way of reducing workflows and speeding up detections in investigations.
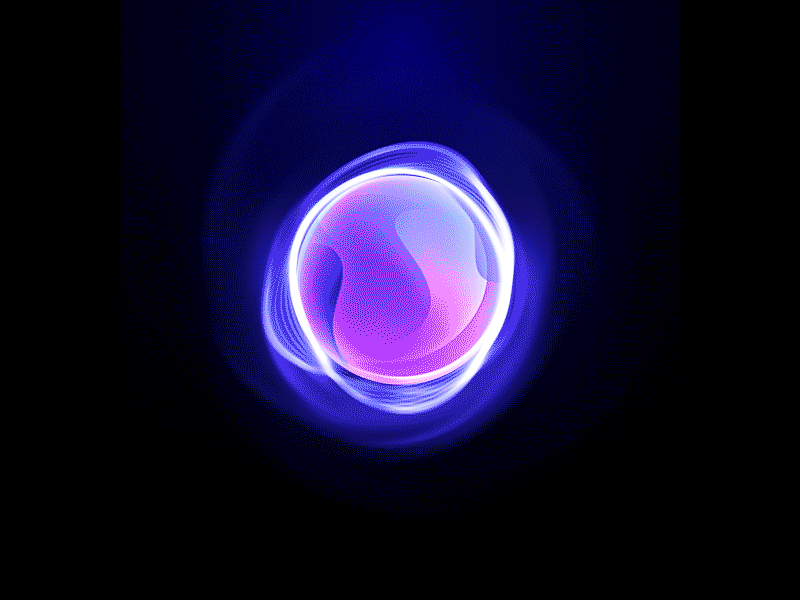
The possibilities for the application of artificial intelligence (AI) seem endless. An app that can identify nearly any piece of music? Check. An algorithm that can evaluate heart disease risk? Check. It seems that the limit to what AI can do is our own imagination.
This article is the first in a series on the subject, I plan to speak to OSINT specialists and find out where the low hanging fruit for AI and algorithmic based tools is, in an attempt to predict how we can best leverage emerging technologies in OSINT.
In this article I approach the subject from a hypothetical perspective, because despite looking far and wide for a good example, there just aren't any yet.
With versions of AI creeping into almost every industry, from music to medicine, how can AI shape open-source intelligence (OSINT) collection? What is the relationship between AI and OSINT, and how can OSINT analysts harness AI to their advantage?
Artificial intelligence (also called machine learning) is essentially machines doing tasks that are normally reserved for humans, such as “visual perception, speech recognition, decision-making, and translation between languages.” There are different types of AI algorithms, from regression (using previous data to predict future data in a graph) to the attempt to replicate human neurons known as artificial neural networks.
Artificial neural networks (ANN) are networks of artificial nodes (the neurons). They are usually made up of three layers: the input layer, the hidden layer, and the output layer. The input layer is the input data, while the hidden layer is where the data is processed and the output layer is the end result.
These systems can identify future examples by generating identifying characteristics from the previous instances. For example, an ANN might differentiate between an image of a cat and an image of a dog by using previous images to give cats a set of characteristics, like whiskers, pointy ears, etc… Then, the ANN can weigh those characteristics against any new images to categorize the image into “cat” or “not a cat”.
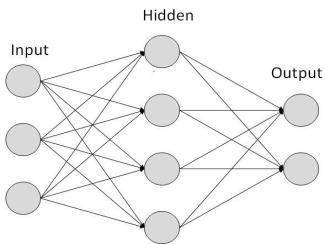
AI could be used for image recognition in OSINT. If an analyst were investigating a video of a war crime and there was a specific weapon used but the analyst didn’t know what kind of weapon it was, an ANN could identify the type of weapon used by comparing it to millions of other images that feature weapons with similar characteristics. This kind of automation is hugely important, because while there are some very talented analysts out there (working for Bellingcat say), there are only so many of them and the workloads they carry is often insane. Being able to offload basic image recognition and pattern detection to AI would be a godsend.
But doesn’t that sound like reverse image search? Yes, but using AI is more accurate and doesn’t rely on the pixels of an image. Reverse image search engines, like TinEye, take the image and assign it a unique “fingerprint”, which is based on the image pixels, and store that fingerprint in a database. So when one uploads an image, the engine gives that image a fingerprint, and checks that fingerprint against the existing database.
With AI, the algorithm wouldn’t rely on image pixels, which makes AI more accurate, since relying on pixels only produces results that are similar in pixel count to the previous image. The search engine wouldn't necessarily deliver results that are similar in terms of image content. But since AI can identify image content, it can be used for more accurate image recognition.
As OSINT becomes increasingly automated, AI will also play a role in collecting data. Already, intelligence agencies globally are using AI to “collect social media data”. According to OSINT expert Nihad Hassan, author of OSINT Methods and Tools "Government bodies, especially military departments, are considered the largest consumer of OSINT sources. The huge technological developments and widespread use of the Internet worldwide have made governments a huge consumer for OSINT intelligence. Governments need OSINT sources for different purposes such as national security, counterterrorism, cybertracking of terrorists, understanding domestic and foreign public views on different subjects, supplying policy makers with required information to quantities of data on the Internet. The act of mining OSINT data by governments is expected to intensify as we move steadily toward what is now a digital age."
All of this points towards enormous amounts of data being collected, data which then must be collated and correlated into some sort of context and in my view this represents some of the essential work that AI could do in order to lighten analyst workloads.
Of course, AI is an emerging field, and image recognition and data collection are just a few of the possibilities for AI and OSINT. As AI grows, the opportunities for AI application in OSINT will only grow too. AI is coming to the OSINT field; in fact, it may already be here. But are OSINT analysts and investigators ready?
If you are active in the OSINT field on social media, you can expect me to reach out to you over the coming weeks to talk to you about this subject. I want to learn where we are with algorithmic image detection and machine learning in OSINT, I also want to compile a list of the tools that can be automated by AI today so that I can share them with you all. If you have any tips, reading suggestions or guidance around this subject, I would love to hear from you!
Just follow me on Twitter and send me a DM!
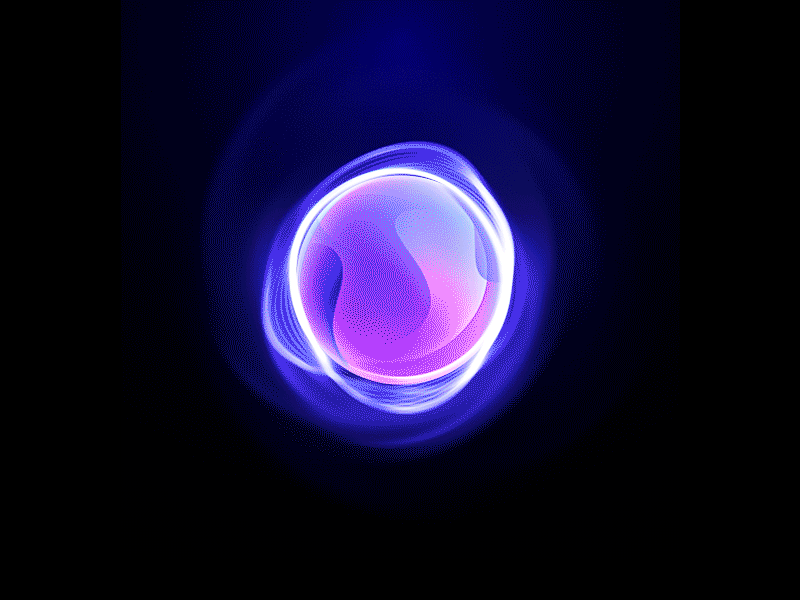